Project Partners
SANTER Reply (capofila), Politecnico di Torino, FCA Items S.p.A., SKF Industrie S.p.A., FERRERO S.p.A., AURORA S.r.l., Farmaceutici PROCEMSA S.p.A. IcxT, Network Integration & Solutions S.r.l., Net4Partners S.r.l., MODELWAY S.r.l., Pro Logic Informatica S.r.l., Filippetti S.p.A., SSB Progetti S.r.l., Ivrea Sistemi S.r.l., Exemplar S.r.l., CEDEO.net, Soft-in S.r.l., ZIRAK S.r.l., Bsim S.r.l., ILLOGICX S.r.l., Netsurfing S.r.l., BLUE Engineeting S.r.l., Al.MEC S.r.l., AMET S.r.l., I.S.M.B., UniTO – DipINFO, TELECOM Italia S.p.A.
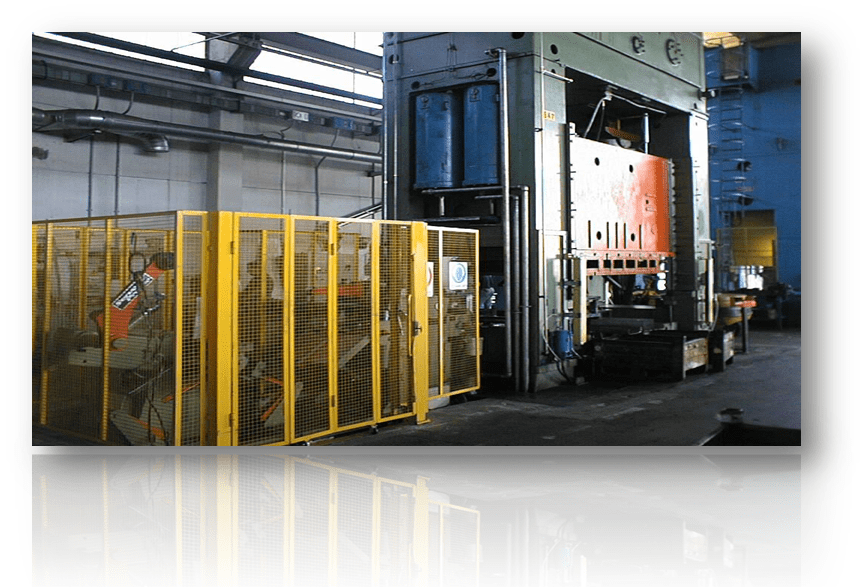
Main tasks
This project has been performed by means of the co-financing of POR FESR Piemonte 2014 – 2020, asse I Azione I.2b.2.2, Smart Factory Technologic Platform, with a financed amount of 73.500,00 €.
The main goal of the project was to design an ICT platform for the integrated, dynamic and autonomous management of production operations with a high level of automation, in order to optimize resources (people, materials, production systems, etc.).
The DISLOMAN platform consists of several hardware and software horizontal modules, applicable to undifferentiated production areas, and vertical modules applied to four demonstration production chains with a high impact on the territory and with different production volumes, sizes and complexities: car manufacturing, machine tools manufacturing, precision mechanics, pharmaceuticals and agri-food.
The platform is based on Internet-of-Things (IoT) technologies and it is characterised by modularity, interoperability and generality.
AMET Tehnical Contribution
AMET has made available to the project its analytical modeling capacity for the simulation of complex objects and systems, such as moving machinery or operating vehicles or their parts, with the aim of carrying out diagnostic assessments aimed at predictive maintenance, through the use of Machine Learning techniques and Artificial Intelligence algorithms.
The project offered AMET the opportunity to approach innovation issues in automation in the manufacturing 4.0 field and to apply the skills in the control field to on-condition and predictive maintenance processes and remote reconfiguration of machinery and plants, in context of the activities aimed at optimizing the production process, on which AMET usually operates.
Activity on prototype
The objective of the activity carried out on the Cellino demonstrator was to identify an optimal maintenance frequency for an ERFURT 800t press, according to the following scheme.
A Machine Learning algorithm was used, trained to recognize a critical operating condition, as a function of the sensible quantities detected and simulated, by means of a numerical model of the machine.
The architectural scheme provides for the transformation of the raw data present in the shop floor into certified, integrated and homogeneous information in accordance with the IoT paradigm: data from machines and sensors are made homogeneous for the various application and service levels.
Routine maintenance is carried out on the machine on a regular basis; Extraordinary maintenance is required in the event that any component breaks, with consequent machine downtime.